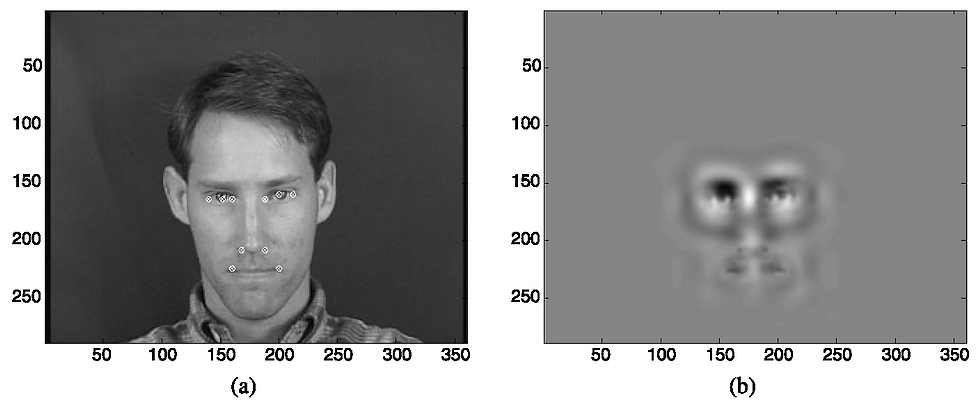
Gabor Filters For Face Recognition Crack + Product Key Full [Win/Mac] [Latest-2022] Face images are usually characterized by many variations and often contain no clear, repeating pattern. To mitigate this, we use Gabor wavelets to search for local feature points in the face images and we extract one set of feature points as the initial landmarks and construct filter bank to extract facial features. Then combine all those feature points in each filter bank and construct a feature vector consisting of Gabor coefficients for each feature point in the face. Finally, identify the face by using the linear discriminant function. The selective search technique from Harris and Stephens is based on local features (in this article, the most active area is the so-called selective search technique from Harris and Stephens is based on local features (in this article, the so-called Selective Search“ technology used in our method, where the important point is that it is a method based on local features, not global matching, and the most active area is the so-called Selective Search” technology used in our method, where the important point is that it is a method based on local features, not global matching, and the so-called the final matching. Method 1. The most active feature point combination search, which search for a feature point combination based on features in each searching step, each of the most active feature point combination search. The specific algorithm is as follows: Start with a small number of feature points, and search for the combination of points that are most effective to the linear classification boundary. The number of points is chosen by the features dimension in the problem; That is, we assume the number of dimensions is fixed. The combination of points, n is chosen by a simple search on a small square grid over a region that surrounds the points (n + 1) for each dimension of the features. The worst-case complexity of this approach is O(2n). The best-case performance is obtained when the combination of points is optimal and its complexity is O(n). The procedure is: Identify candidate points by using a fixed set of filters at a fixed location in the face image. From the n candidates, find the best combination by searching for the combination of points which is most effective to the boundary. The feature point combination and Gabor coefficients are the two primary components of the feature vector. Method 2. Harris–Stephens, which searches for the most effective number of simultaneous feature points. The specific algorithm is as follows Gabor Filters For Face Recognition Crack+ Latest =============================================== Face recognition has primarily been done using the shapes and sizes of facial features as inputs. Face detection and feature location is the initial step in all face recognition systems. Feature detection typically requires specialized segmentation software, segmented by experts, and the faces need to be properly aligned (or warped) to a coordinate system. Even with a perfect face detector and alignment software, it is not always possible to detect facial features. There are two reasons for this. First, the features are not always well-aligned with the coordinate system. Second, the focus of face detectors is very much on facial features (which is all that most face recognition requires) and not on finding the edges of the face. What is typically needed is a detector that is good at extracting all the facial features, especially the eyes, even if that detector is not perfect. Face detectors can be done, (and in fact have been done), both by software and hardware. However, all face detectors have drawbacks when it comes to robustness. In order to be robust, a face detector must be particularly good at distinguishing faces from other objects in the image, like say, a car or a tree. As a consequence, robust detection is often done using a number of alternative detectors to the usual eye detector. Also, the detectors should be able to detect faces in the presence of many other faces, backgrounds, distractions, lighting, distortion, and the like. A face detector that is not well-aligned with the coordinate system of the face will produce false positives in the form of incorrect detection of features (the most common example being the detection of eyebrows, hair, or the mouth). An unsatisfactory alignment will also cause misdetection of facial features. Furthermore, the best face detectors are too slow to be used for face recognition. As a result of these problems with face detection, the “average” face detector is not very good at finding features on faces, especially small features like the eyes. Hence, the feature locations are not as easy to locate with a good face detector as they would be if the face is properly detected. This is especially true for faces that are extremely dissimilar from each other. There have been a number of proposals for face feature location, some of which use information from the corner coordinates of a face. One paper, for example, uses the eye coordinates (a given number of pixels from the left and top of the eye) to determine the location of the eye. Another technique uses a combination of corner coordinates and a hist 09e8f5149f Gabor Filters For Face Recognition With License Code For PC The Gabor filters are a variant of the Gaussian function that supports localization and invariance properties. They were introduced by D. Gabor in 1946 as the impulse response in the framework of a visual system. While the Gaussian function is used to describe the complex spatial probability density function of the spatial distribution of edge-detectors, the Gabor function is used to describe the probability density function of a localized edge detector. Both are components of the visual system, in that the Gabor-based detectors are central vision components with an exact location of the detected edge-pixels. We know that the human visual system can detect color, brightness, contrast and edge-detectors, but still cannot detect size or distance.\ A Gaussian function is defined as, $$\begin{aligned} G(x,\sigma,\rho)=\frac{1}{\sqrt{2\pi \sigma^2}}e^{\frac{ -x^2}{2\sigma^2}}e^{\frac{i\rho x}{\sigma}},\end{aligned}$$ such that $x=0$ is the center of the function. This function represents the probability density function of a complex random variable, and it peaks when the modulus of the argument of the exponential function is equal to $\rho/\sigma$ (Figure \[golalbafigure\]). A Gabor function is defined as, $$\begin{aligned} G(x,\sigma,\rho)=\frac{1}{\sqrt{2\pi \sigma^2}}e^{\frac{ -x^2}{2\sigma^2}}e^{\frac{i\rho x}{\sigma}}e^{ -\frac{x^2}{2\sigma^2}},\end{aligned}$$ such that the center of the function is $x=0$ and the width of the function is $\sigma^2$ (Figure \[golalbafigure\]). In our case $\rho$ represents the orientation of the function and $\sigma^2$ the length of the function. If we define the Gabor function as $G(x,\sigma,\rho)e^{i\rho x}$ and we separate the real and imaginary parts by multiplying it with $e^{ -i\rho What's New in the? --------------------------- In this section, we will look at the details of our Gabor filters approach to Face Recognition. Local feature-based detection and recognition are a very powerful and effective approach in face recognition. Compared to vector-space face recognition, where training sample images are available, face feature-based algorithms can be used even for identifying face images that have been captured from a surveillance camera or on the web. Face images contain many generic features (known to be present in all faces) which can be used to uniquely identify the face. Typically, the features are based on intensity distribution, which fall into two major categories: local texture features [@glens] and morphological features [@Bishop]. For face recognition purposes, we only need to extract features in a small region around the eyes and mouth, as these are the generic features that are invariant to pose variations. In order to extract such features, we use a Gabor filter to map the image in the frequency domain. Gabor filter is a filter bank that consists of many sinusoidal planes (Gabor wavelets) that are tiled over the input image. The input image $f$ is convolved with the Gabor wavelet $g(\omega,\beta )$: $f\otimes g(\omega,\beta )$: $$g(\omega,\beta )=\exp (\frac{ -\beta }{2}\vert \omega \vert ^{2})$$ where $\omega $ is the spatial scale of the filter, $\beta $ is the scale of the sinusoidal wave. In general, the Gabor filter can be applied to an image in either of two ways. The first method is the multi-scale ‘shift and scale’ algorithm, where the input image is convolved with a Gabor filter at each pixel, and the result is shifted and scaled to its original location. In the second method, we first compute Gabor filter responses for the desired spatial frequencies. Then, the Gabor filter responses are applied directly to the original image. Each pixel of the resulting image is a weighted sum of all the Gabor filters at the desired frequency. As we know, the central problem in computer vision is to efficiently and effectively locate distinctive features (edges, corners, etc.) in a face image. This problem is usually divided into two parts: First, we need to find the locations of characteristic points (feature System Requirements: Terrain: hills, mountains, and random mountain huts; large stone and wood pillars scattered throughout World: world 1, mountain 1, mountain 2, mountain 3, world 2, world 3 Character Creation: level 1-3 Racial Level: level 1-3 Attributes: level 1-3 Recruiting Tools: General Recruiting: 2-5 Mountain Snipers, 5-10 Fiddlers 6-10 Mountain Strikers, 6-10 Fiddlers
Related links:
Comments